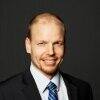
AI without machine learning
Dr. Frank Sauberlich discusses how AI can exist without machine learning.
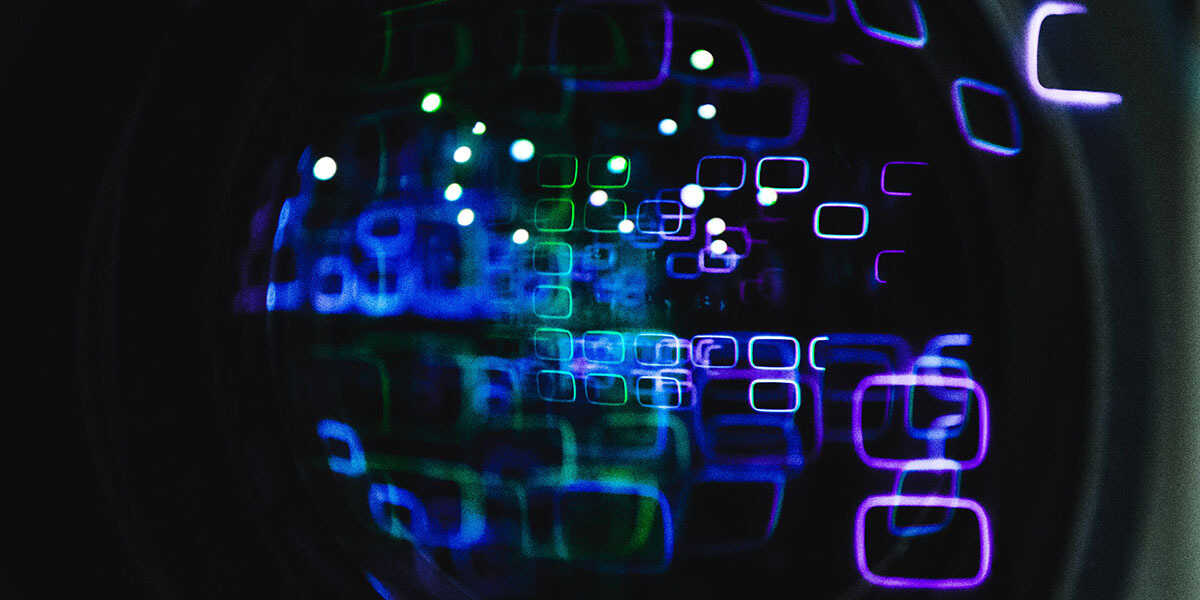
Previously, we explained how machine learning can have its own life and doesn’t need to be a part of AI. Here, we want to explain something that may surprise you: it is possible to build AI without machine learning. “A car with no engine!?” you may cry. Analogies are made to be broken.
There are several companies that do exactly this: deliver AI that does not rely on machine learning.
This may sound like a contradiction. The very heart of AI is machine learning, right? Actually, this is not true. Historically, artificial intelligence preceded machine learning. Researchers have found ways of creating AI without even knowing about machine learning. And these “ancient” ways of creating AI are still alive and well, and used today more than ever.
As a starter, the chart below shows the usage of the terms artificial intelligence and machine learning over time. Artificial intelligence seems to have taken off as early as 1950. By contrast, machine learning was not commonly used before the late 1970s. That corresponds to a 20-year lag. Wow! Twenty years of AI without machine learning. And in the 1980s, when machine learning began to be widely used, the term artificial intelligence had an exponential upsurge.
Interestingly, the term artificial intelligence experienced a hard reduction in use after the explosion of the ’80s. This was called the AI winter (we do not seem to have had a machine learning winter). Look here for more discussion on how to reduce the risk of running into an AI winter again.
But how is this possible? How could AI have existed without machine learning? How could people have written about AI without mentioning machine learning? Or did machine learning algorithms exist back then, but were called something different?
In a previous post we saw the two ways to endow machines with knowledge: direct by humans, or indirect by machines learning on their own. Therefore, if there was no machine learning in the late ’50s and ’60s, what were people doing?
If you insert a small amount of knowledge into a machine, you can call it an engineering product. But if you instil a sufficiently large amount of knowledge such that the machine makes better decisions than a human, what do you call it then? For example, if you take hundreds of medical doctors and each spends hundreds of hours detailing correlations between symptoms and disease, you have created something impressive. What if you then pack that knowledge into an easy-to-use machine, which will output a likely diagnosis based on the symptoms you input? Is that an AI?
Well, yes, it is. In fact, today this type of AI we sometimes call GOFAI – an acronym which stands for “good old-fashioned AI”. GOFAI was based on a human-understandable symbolic system. It is an AI without machine learning.
A car with no engine
Let’s go back for a moment to our engine/car comparison. GOFAI is like a car without an engine, like carriages, carts, cars suspended on cables, and so on — all of which existed before steam, combustion, or electric engines were invented.
But is GOFAI still relevant today? Doesn’t it belong to history? Isn’t it the case that all AI today is based on machine learning, and expert systems are so inferior to machine learning that nobody uses them anymore?
Not at all. In fact, GOFAI is used today much more than you realise – certainly much more than media coverage would suggest. Behind all the hype and excitement of machine learning and creating a complete AI, there is almost always some GOFAI in the background. In one form or other, GOFAI is a necessary part of an AI solution to get the full job done.
GOFAI is used in two ways today:
1) Supplementing the work of machine learning in creating a full AI product
2) Producing an AI solution on its own, without any machine learning
As we discussed in the first post of this series, a complete AI needs parts in addition to machine learning. A good chunk of these ‘additional parts’ are GOFAI parts.
Almost every AI product that you see today needs content inserted directly by human experts. This may be expertise harvested from linguists and phoneticians if the AI is using natural language processing, from physicians in cases where the AI is used in medicine, or perhaps even from experts in road traffic and driving when the AI powers self-driving cars, and so on. Machine learning couldn’t create a full AI without the assistance of GOFAI components.
Just as a toaster contains human-inserted knowledge on the typical size of a slice of bread through its design, AI solutions are always built with knowledge given by humans – often in some implicit way. Importantly, these GOFAI parts are extremely useful as they can help machine learning do its learning job much quicker by providing appropriate inductive biases. That way, GOFAI empowers machine learning algorithms to make their own learning decisions.
Pure GOFAI
In the present age of flourishing machine learning and teraflops of operations in GPUs and TPUs, can a company remain competitive if they create products that use nothing but expert systems fully specified by human-given rules? The answer is again affirmative.
There are specialized companies and great products that are solely based on GOFAI. For example, one company produces an AI solution to govern computers across the organisation. Tickets are raised each day by the tens of thousands of employees at the company, from requesting more hard disk space, to fixing broken email clients, to password change requests. Much of these activities are simple routines, so an AI is employed to perform a good chunk of that work automatically, first by feeding the immense knowledge of technicians into a machine, then letting the machine fix the common issues. Human effort can then be redirected to solve only the new, more complex issues. This is done at Arago.
Another common use of GOFAI are chatbots. Today, despite the immense progress of natural language processing through machine learning, many of the chatbots that you may encounter are solely based on GOFAI. Within a limited domain, such as assisting customers with a single service, a set of human-defined rules on how to reply to customer questions can go a long way.
Hence, the next time you encounter a chatbot, you may not only ponder deep, philosophical questions regarding a Turing test – can a machine ever make us believe it is a human? You may also question which generation of AI is behind the chatbot: is it good-old-fashioned-AI or machine-learning-AI?
In conclusion, not only can machine learning exist without AI, but AI can exist without machine learning.
Now, can we define AI and machine learning precisely enough to avoid future confusion? This question will be addressed in our next (and final) post in this series. Stay tuned.
Find out more about AI for the enterprise: https://www.teradata.com/Insights/Artificial-Intelligence.
알고 있어
테라데이트의 블로그를 구독하여 주간 통찰력을 얻을 수 있습니다