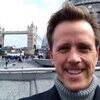
How to Get More ROI—Faster—From Machine Learning
Find out how to harness machine learning and AI to contain costs, increase revenue, and grow your organization’s customer base. Read more.
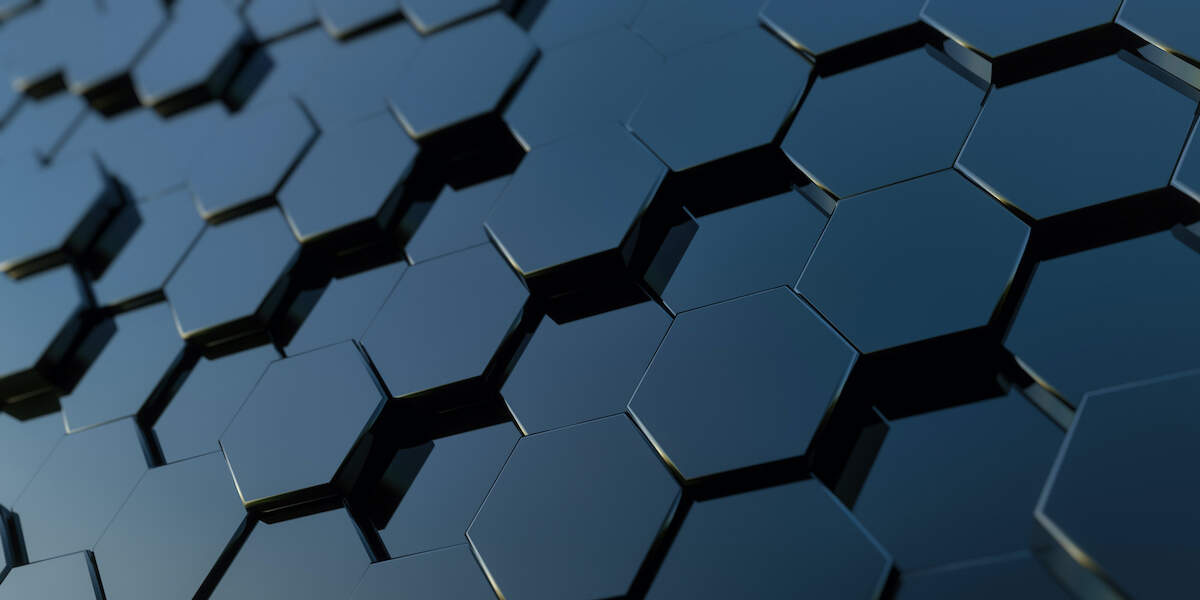
In 2016, Gartner’s Hype Cycle rated machine learning and AI “the most disruptive class of technologies.” Companies were quick to incorporate the promising capabilities into their advanced analytics efforts.
But the technologies haven’t delivered real value for most organizations.
Senior executives tell McKinsey they’re “eking out small gains from a few use cases” and “failing to embed analytics into all areas of [their] organizations.” And Gartner has since estimated that over 80% of machine learning projects fail to reach production.
Why have machine learning and AI failed to deliver?
Two barriers holding back your analytics
The traditional approach to analytics—per-application custom data feeds, multiple data copies, and nine months or more for implementation cycles for a single model—doesn’t work for analytics at scale.
To succeed at scale, enterprise analytics programs need to overcome the two largest barriers to ROI: scale of analytics and scale of data.
Barrier #1: Scale of analytics
Machine learning algorithms perform best when given tasks that are discrete and specific. As the size of the problem space becomes larger and more complex, single models fail to perform.
Case in point: A child’s scooter, a wheelchair, and a city bus all have wheels, but each operates very differently. A self-driving car needs to understand the differences between these “vehicles”—while also knowing how to detect and behave at red lights, stop signs, and other traffic signs. A single model approach can’t manage that complexity at scale.
The solution? Break the problem space into small units and deploy machine learning at the lowest level possible. Future-ready businesses will need hundreds of thousands—to millions—of algorithms working together. Hyper-segmentation—where a single algorithm is trained against each customer’s experience and data, instead of a single algorithm trained against all customers—will be necessary in some cases.
Barrier #2: Scale of data
Why does Google always—and eerily—know what you’re about to ask it?
Data.
Google has amassed trillions of observations from billions of daily searches—plus millions of data points from interactions with individual users. For machine learning to perform well, enterprises must use all their data. That includes data from across the enterprise, across products, across channels, and from third-party data vendors.
However, we don’t just need more data. We need more data in context—cataloguing it by party or organization, by network, by time, and with geospatial and biometric overlays. Pennies, Pounds, Euros, and Rupees are all names for money, but we don’t want machine learning to try and understand each currency. We want it to understand credit risk, predict probability of a large loss, or determine optimal inventory levels.
Data in context also needs to be relatively clean, so it can be well understood by all users—including the analytics algorithms, end users, auditors, senior executives—or worst-case scenario, opposing counsel.
Building reuse, flexibility, and ROI into analytics at scale
Consider this:
- Data processing accounts for 80% of any given project’s time expenditure
- Close to 65% of the processed data can be shared, even in remotely similar use cases
- Leveraging this data can save organizations hundreds of thousands of hours
But traditional solutions require a time-intensive process of copying and moving data to each application.
That’s why scaling analytics isn’t just a matter of investing more money in analytics but investing in the right data management design. To be future-ready, organizations require a connected multi-cloud data platform to cut through complexity and deliver useful, actionable answers to any problem.
Find out how to harness machine learning and AI to contain costs, increase revenue, and grow your organization’s customer base. Sign up for our Analytics 1-2-3 webinar.
알고 있어
테라데이트의 블로그를 구독하여 주간 통찰력을 얻을 수 있습니다