How Tesla is Redefining the Auto Industry
New players like Tesla are changing the automotive industry into a software-driven paradigm which has made data management & analysis at scale a critical capability for OEMs.
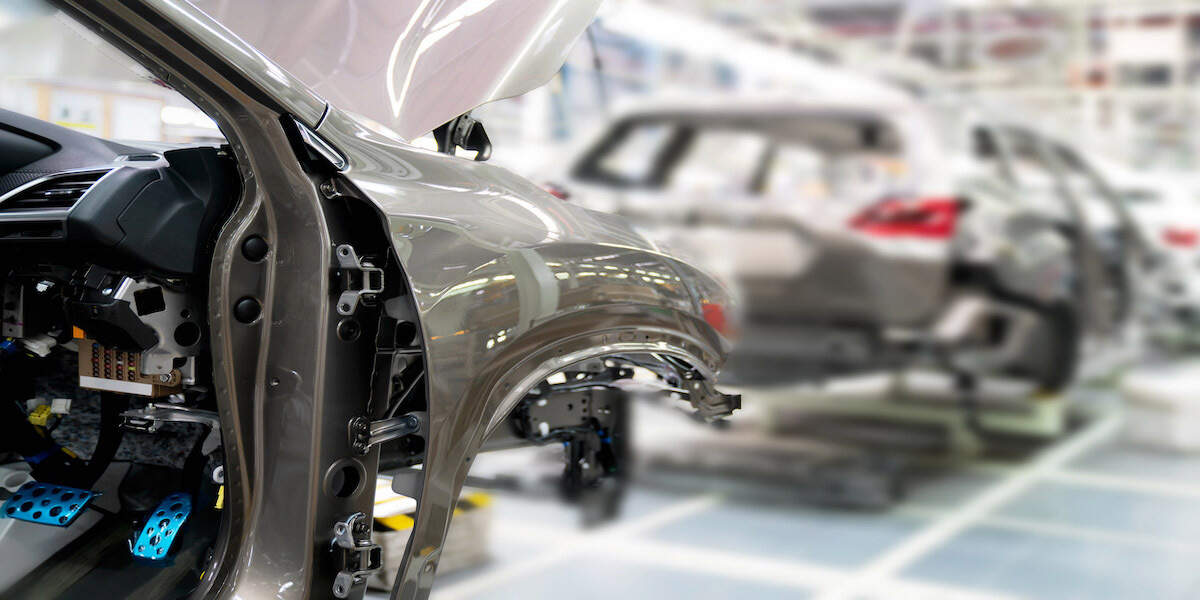
The new paradigm shift in the auto industry
Tesla is not a typical automaker. In most ways it closely resembles a Silicon Valley technology start-up. Traditional Auto companies, “the metal benders,” are adept at making complex reliable products at massive scale, but the software experience in those products is still not as sophisticated as we experience every day with our mobile devices, gaming consoles, smart wearables etc.
Very soon, we may see well known Auto brands – the likes of VW, Toyota, FCA, GM -- join the list of Silicon Valley technology companies.
But why?
Why can’t auto companies focus on building good cars and let their tech partners/suppliers build the software for the cars?
The reason for this paradigm shift in the auto industry is the competition from clean sheet companies who are changing the rules of the game - Tesla, Lucid, Revian. They have embraced technology at their core. This has completely changed the relative importance of software vis-à-vis hardware in a vehicle. The customer and market acceptance of this new future paradigm is also reflected in the valuations of companies like Tesla (see figure below).
Traditional cars start getting degraded from the day they are driven out of the showroom -- it is a downhill road. Companies like Tesla are improving the performance of their cars over time by intelligently using the data collected through frequent over the air updates (OTA). One recent example is Tesla increasing the performance of its Autopilot system significantly over a period of 18 months (see figure below). The Autopilot performance almost doubled in the period of 18 months, whereas the performance of active safety features remained almost the same.
This is their true edge; the software core turns every Tesla car into a learning machine. Tesla’s global fleet of cars have collected data of 3bn miles, have identified multiple edge scenarios and done over 120 OTA updates since 2017 -- that’s an average of one update every 16 days. These updates have added new features like Smart Summon (valet service), added performance like additional 40 BHP to Model-S, added support for new content like Netflix, and fixes and updates to systems like BMS, braking, Autopilot, etc. This is a major competitive advantage. For other car companies, these would have meant expensive and messy recalls and updates, or may not even be possible in the existing vehicle platforms.
This concept of products getting better over time is not at all new – we are used to it in our smart phones, smart TVs, tablets, PCs. They add functionalities, content, bug fixes, etc., through updates. However, this concept was alien to the Automotive industry until recently; almost all car models deteriorate from the time they roll out of the show room. Also, these products are disconnected from our digital life, unlike our other smart devices.
The ship is turning
The ICE industry has grown by outsourcing subsystems, including software and the processing compute hardware. Some of the major OEMs have outsourced nearly 90% of the software which goes into their cars. To make software and data analytics the core of the industry, there are calls for a significant change in the way the OEMs function.
This is not lost on the major Auto players. Some of the major Auto giants like Volkswagen and others have made commitments to build software and data analytics at their core. Companies like VW, GM, Toyota, Hyundai, FCA are making large investments towards making this shift. But it is a major leap ahead for traditional Auto companies. There needs to be a significant shift in culture, skills, processes and many more of the legacy aspects of the automotive industry.
The vehicle design and manufacturing process has generally been quite linear with a typical lead time of 2-3 years for a new product, and there isn’t much learning built into the vehicle. The software development process is quite different. It has a built-in mechanism for collecting vast amounts of data from the products in the field to capture the edge cases and to better understand how the product is being used in the field. It can uncover newer requirements and continually improve the product while in use. This process of continuous improvement i.e., learning by collecting large amounts of data and improving the product. is baked into everything from a small mobile game to the largest software platforms on the planet, from mobile phones to large industrial machines. It also needs to be an intrinsic part of the automobiles of the future.
The Data Conundrum and the Secret Sauce
This calls for appreciating a very fundamental issue. The scale, speed and complexity of data from the vehicles and the need for analysing that data in conjunction with the enterprise data of BoM, customer master, service history, etc. A typical ADAS equipped car would generate roughly 1TB of raw data per day. A vehicle fleet of 100 thousand such cars would generate 100 petabytes of raw data per day! That’s a lot of data to store and analyse. This requires the ability to manage data at extreme scale in a reliable manner.
Also, there are decisions to be made regarding the following: which data to extract and store, what is the cost of storage, who gets access to which data, what will be the design of the data transfer pipeline, what advanced analytical algorithms can be used, which architecture will perform at such scale, which platform has the ability to handle unstructured and semi-structured data and many more.
There is a need to architect and build a data pipeline that can manage exabyte scale data. A data pipeline that can collect and intelligently sift out important data elements and then link those with enterprise data for the analysts to work on using advanced pattern recognition and other machine learning and AI techniques. Managing this data deluge intelligently will be a critical capability for OEMs to succeed in this race.
The OEMs need to partner with a data and analytics organization that understands the complexities of operationalizing hyperscale architectures to support critical business challenges at scale. Teradata has been helping organisations make sense of sensor data at massive scale, and to use that to solve complex business problems and create future-ready capabilities.
알고 있어
테라데이트의 블로그를 구독하여 주간 통찰력을 얻을 수 있습니다